Harnessing Machine Learning in Agriculture: A Comprehensive Guide to Agriculture Datasets
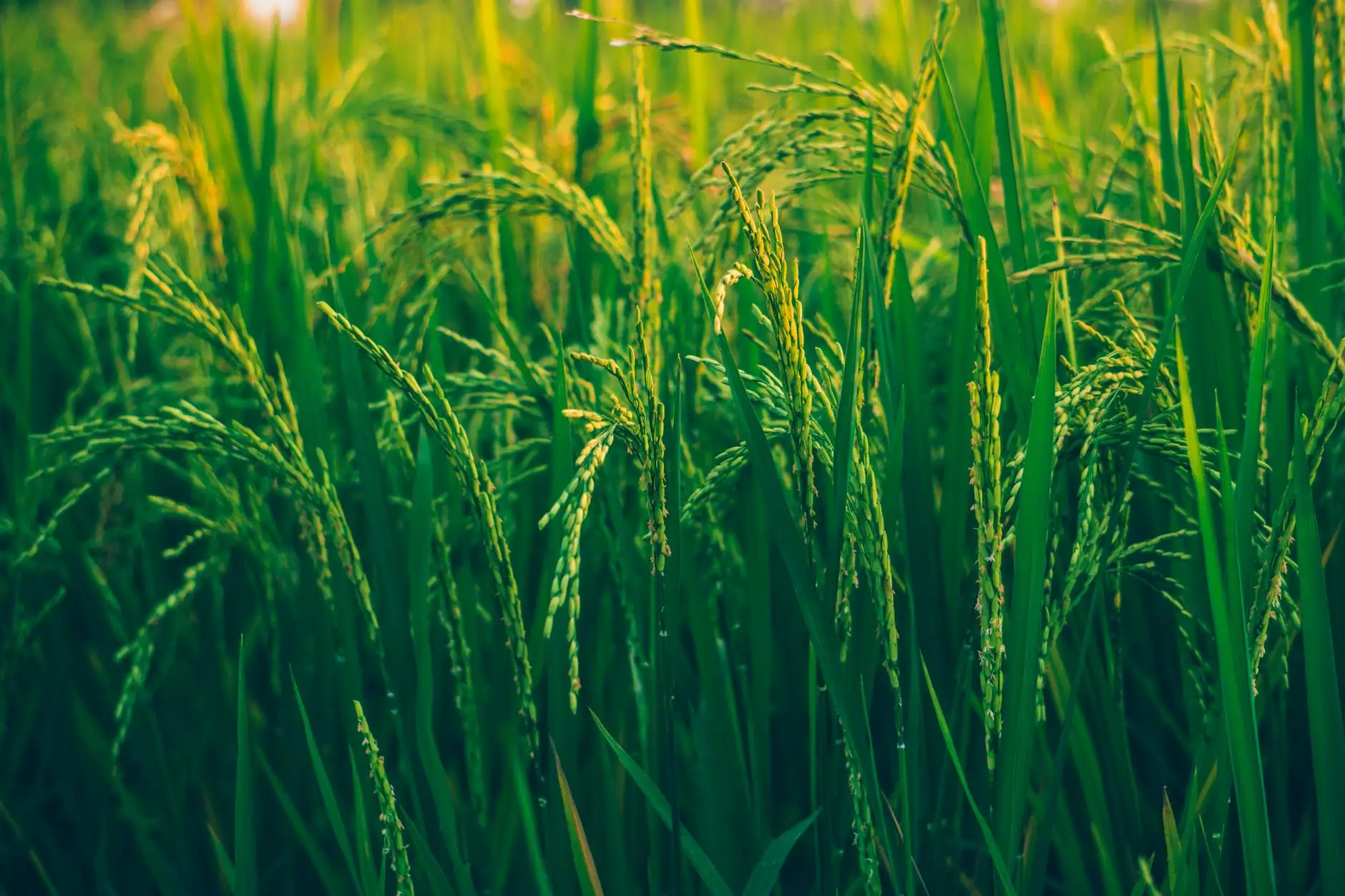
The intersection of agriculture and technology is witnessing a phenomenal transformation, primarily driven by the advent of machine learning (ML). One of the most critical components enabling this change is the availability of robust and relevant agriculture datasets for machine learning. This article delves deep into understanding these datasets, their significance in enhancing agricultural practices, and how businesses can leverage them to drive innovation and improve productivity in the Home Services, Keys & Locksmiths sector.
Understanding Agriculture Datasets
In the ever-evolving field of agriculture, data plays a crucial role. Agriculture datasets consist of a myriad of information related to farming practices, crop yields, soil health, weather patterns, and market dynamics. These datasets are pivotal in machine learning as they provide the essential raw material that algorithms need to learn and make informed predictions.
Types of Agriculture Datasets
- Crop Yield Data: Information on the yield of various crops over different seasons and conditions.
- Weather Data: Datasets that include temperature, rainfall, humidity, and climatic conditions affecting agriculture.
- Soil Data: Contains data about soil composition, health, and fertility levels.
- Pest and Disease Data: Information on outbreaks, pest behavior, and disease impact on plant health.
- Market Data: Trends, prices, and demand-supply information that affects agricultural products.
The Role of Machine Learning in Agriculture
Machine learning has ushered in a new era for agriculture, where farmers and agricultural businesses can utilize data-driven insights to make better decisions. Machine learning algorithms process large volumes of diverse datasets to uncover patterns, optimize operations, and predict outcomes.
Applications of Machine Learning in Agriculture
Here are some significant applications of machine learning in the agricultural sector:
- Precision Farming: Utilizing data analytics to decide the optimal planting times, fertilizer application, and pest control measures.
- Crop Monitoring: Leveraging drones and satellite imagery, combined with ML algorithms, to monitor crop health and growth.
- Predictive Analysis: Anticipating crop yields and market trends by analyzing historical data.
- Anomaly Detection: Identifying abnormal patterns such as pest infestations or soil degradation through data analysis.
- Irrigation Management: Implementing smart irrigation systems that use predictive models to optimize water usage.
Obtaining Quality Agriculture Datasets for Machine Learning
Accessing high-quality agriculture datasets is vital for the success of any machine learning project in this field. Here are some key sources and considerations when obtaining these datasets:
1. Public Datasets
Several organizations and institutions provide open-access datasets that can be utilized for research and development in agriculture. Reputable sources include:
- USDA National Agricultural Statistics Service: Offers a wealth of data on various crops and livestock.
- Kaggle: A platform that hosts numerous datasets contributed by the community, including agriculture-related data.
- World Bank: Provides various global agriculture data pertinent for analysis and modeling.
2. Government and Research Institutions
Many government departments and agricultural research institutions publish datasets collected through surveys and experiments. These datasets are often more specialized and can provide deeper insights.
3. Satellite and Sensor Data
With the help of remote sensing technologies, data on environmental variables and crop health can be collected. Organizations like NASA and ESA (European Space Agency) provide free satellite imagery that can be leveraged to enhance agricultural analysis.
4. Agricultural Technology Companies
In recent years, numerous AgTech companies have emerged that specialize in collecting and analyzing agricultural data. Partnering with these companies can provide access to exclusive datasets and innovative tools for data analysis.
Best Practices for Using Agriculture Datasets in Machine Learning
To ensure successful project outcomes, understanding the best practices for working with agriculture datasets is essential:
Data Preprocessing
Data preprocessing involves cleaning and transforming raw data into a usable format. Common steps include:
- Handling Missing Values: Identify and deal with any missing or incomplete entries.
- Normalization: Scale the data to a common range to improve model performance.
- Data Augmentation: Enhance existing data through artificial expansion techniques to prevent overfitting.
Model Selection
Select the appropriate machine learning model based on the type of problem (e.g., regression for yield prediction or classification for pest detection). Some popular models include:
- Linear Regression: For predicting crop yields based on historical data.
- Decision Trees: For making binary or multi-class classifications.
- Support Vector Machines (SVM): Useful in scenarios with complex boundaries between classes.
- Neural Networks: Particularly effective in handling large datasets for intricate prediction tasks.
Model Evaluation
It is crucial to evaluate your model's performance using appropriate metrics such as accuracy, precision, recall, and F1 score. Cross-validation techniques can provide a more robust estimate of how your model will perform on unseen data.
Challenges in Using Agriculture Datasets for Machine Learning
While the potential of machine learning in agriculture is enormous, several challenges persist:
- Data Quality: Inconsistent data quality can lead to poor model performance.
- Data Privacy: Concerns related to data sharing and information ownership.
- Integration of Cross-Disciplinary Knowledge: Successful outcomes often require collaboration between agronomists, data scientists, and software developers.
Future Prospects of Machine Learning in Agriculture
As both the agricultural industry and data science evolve, the integration of machine learning will flourish, revealing new horizons for productivity and innovation. Some anticipated trends include:
- Automated Farming: Increased reliance on AI-powered machines to perform farming tasks autonomously.
- Enhanced Predictive Analytics: More advanced models capable of predicting crop outcomes with unprecedented accuracy due to improved datasets and algorithms.
- Sustainability Initiatives: Implementing ML to reduce waste and improve resource efficiency in agricultural practices.
Conclusion
In conclusion, the explosion of available agriculture datasets for machine learning is revolutionizing how we approach farming and agriculture-related industries. By embracing data-driven methodologies, businesses in the Home Services, Keys & Locksmiths sector, and beyond, can unlock new levels of efficiency and strategic insight. It is imperative for stakeholders to stay ahead of the curve, leveraging machine learning to not only enhance productivity but to also contribute to sustainable agricultural practices globally.
For businesses looking to thrive in this new digital age, a proactive approach to integrating data science into everyday practices is not a choice but a necessity. The future of agriculture is bright, and those who harness the power of *data* through machine learning will undoubtedly reap significant benefits.
agriculture dataset for machine learning